The fintech sector constantly evolves, fueled by the ever-growing need for more advanced and secure fintech solutions. Recent research paints a promising picture for the global fintech industry, suggesting customer growth rates averaging above 50% across industry verticals. Additionally, revenues in the sector are projected to grow almost three times faster than the traditional banking sector between 2023 and 2028.
To keep up with such demands, fintech organizations are embracing new technologies to streamline processes, personalize experiences, and gain a competitive edge. Large language models are emerging as a powerful tool in this landscape. They offer businesses the ability to automate tasks, improve communication, and generate data-driven insights.
Put simply, large language models (LLMs) are a type of artificial intelligence trained on massive amounts of text data. This allows them to understand and respond to human language fluently and accurately. They can perform a variety of tasks like text generation using different creative formats, from marketing copy to financial reports. They can also translate text from one language to another, breaking down communication barriers in the global fintech space. LLMs can answer complex questions posed in natural language, facilitating efficient information retrieval for both internal teams and customers.
Table of Contents
Enhanced Efficiency
LLMs can automate repetitive tasks, such as data entry or report generation, freeing up human capital for higher-value activities. This leads to increased productivity and cost savings. Fidelity Investments, headquartered in Boston, is investigating how LLMs can automate the generation of regulatory reports. LLMs can analyze complex financial data and translate it into reports that comply with specific regulatory requirements, saving significant time and resources for compliance teams.
Improved Customer Support
LLMs can provide 24/7 customer support through chatbots, answer questions accurately, and personalize financial product recommendations. This fosters better customer engagement and satisfaction. Nuvei, a leading payment technology provider, leverages its FinText messaging and payment platform to address customer requests effectively. Their system can handle routine inquiries and transactions, freeing up human agents to focus on complex issues.
SoFi applies a different approach by utilizing LLMs to facilitate a smoother onboarding experience for new customers. LLMs can process identification documents, answer basic questions about account features, and personalize welcome messages – all through a conversational and efficient interface.
LLMs may also be used to automate the drafting and execution of smart contracts. This ensures greater accuracy and efficiency in financial transactions.
More Personalized Services
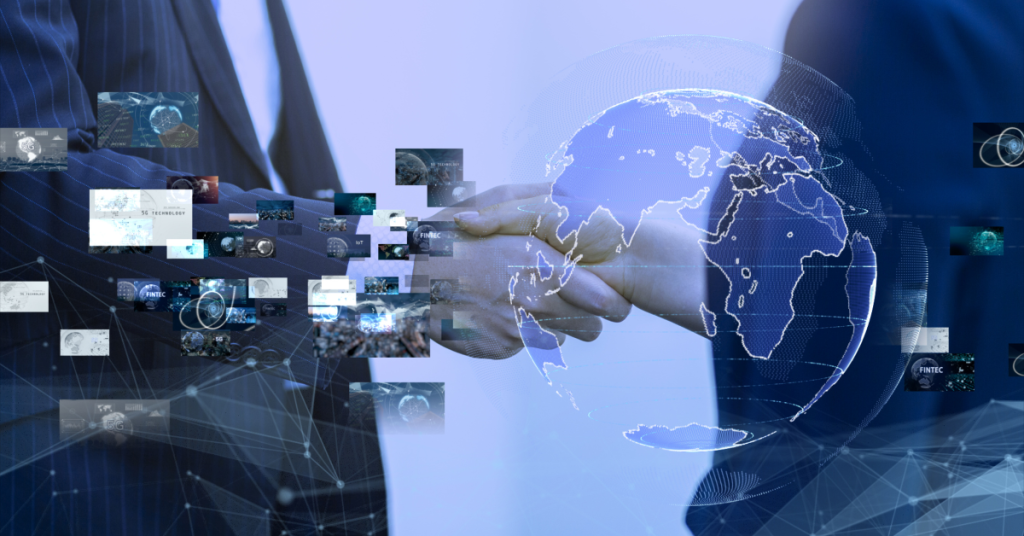
By analyzing alternative data sources beyond traditional credit scores, such as social media profiles or online banking history, LLMs can generate personalized risk assessments. For instance, the lending platform Upstart uses LLMs to develop more nuanced credit scoring models. This helps them paint a more complete picture of a borrower’s financial situation.
Data-Driven Decision Making
By identifying patterns and trends in vast datasets, LLMs can assist traders in making informed investment decisions. Alpaca, a fintech company focused on providing tools for self-directed investors, is exploring the use of LLMs to analyze market data and generate trading signals.
Better decision-making also benefits from having access to personalized financial advice. Charles Schwab is adopting LLMs to generate personalized financial planning content for its customers. This allows the company to provide tailored guidance at scale, catering to individual financial goals and risk tolerances.
Better Security
LLMs contribute to fraud detection by studying vast financial data sets in real-time. California-based Affirm leverages LLMs to analyze loan applications and identify potential fraud patterns. This helps mitigate risk and ensure the financial security of both the company and its customers.
The credit card provider Brex implements a similar strategy by letting LLMs analyze spending habits and identify suspicious transactions. By doing so, it enhances risk management capabilities and protects businesses from financial losses.
These examples showcase the diverse applications of LLMs in the fintech space. As the technology matures, we can expect even more innovative use cases to emerge, transforming how financial services are delivered and experienced.
The Importance of LLMs for Continued AI Development
As more businesses embrace artificial intelligence (AI), LLMs are playing a crucial role in building more advanced models. For starters, LLMs can improve AI learning by generating vast amounts of high-quality training data for other models. This data can be diverse and unbiased, leading to the development of more robust and accurate AI systems.
The ability of LLMs to understand and generate human language is also crucial for advancing natural language processing (NLP) capabilities. This paves the way for more natural and intuitive interactions between humans and machines.
Lastly, LLMs can be used to automate tasks in AI research, such as data analysis and model evaluation. This frees up researchers to focus on more creative endeavors, accelerating the pace of AI development.
Conclusion
The integration of LLMs presents exciting opportunities for fintech businesses. As companies in the sector continue their adoption, they can better streamline operations and enhance customer experiences. These tools allow organizations and clients alike to make data-driven decisions for growth and success.
[…] HRMS Globex’s focus on these features highlights its dedication to providing all-encompassing HR management solutions tailored to modern business needs. […]
[…] Also Read: Large Language Model Integrations in Modern Businesses […]